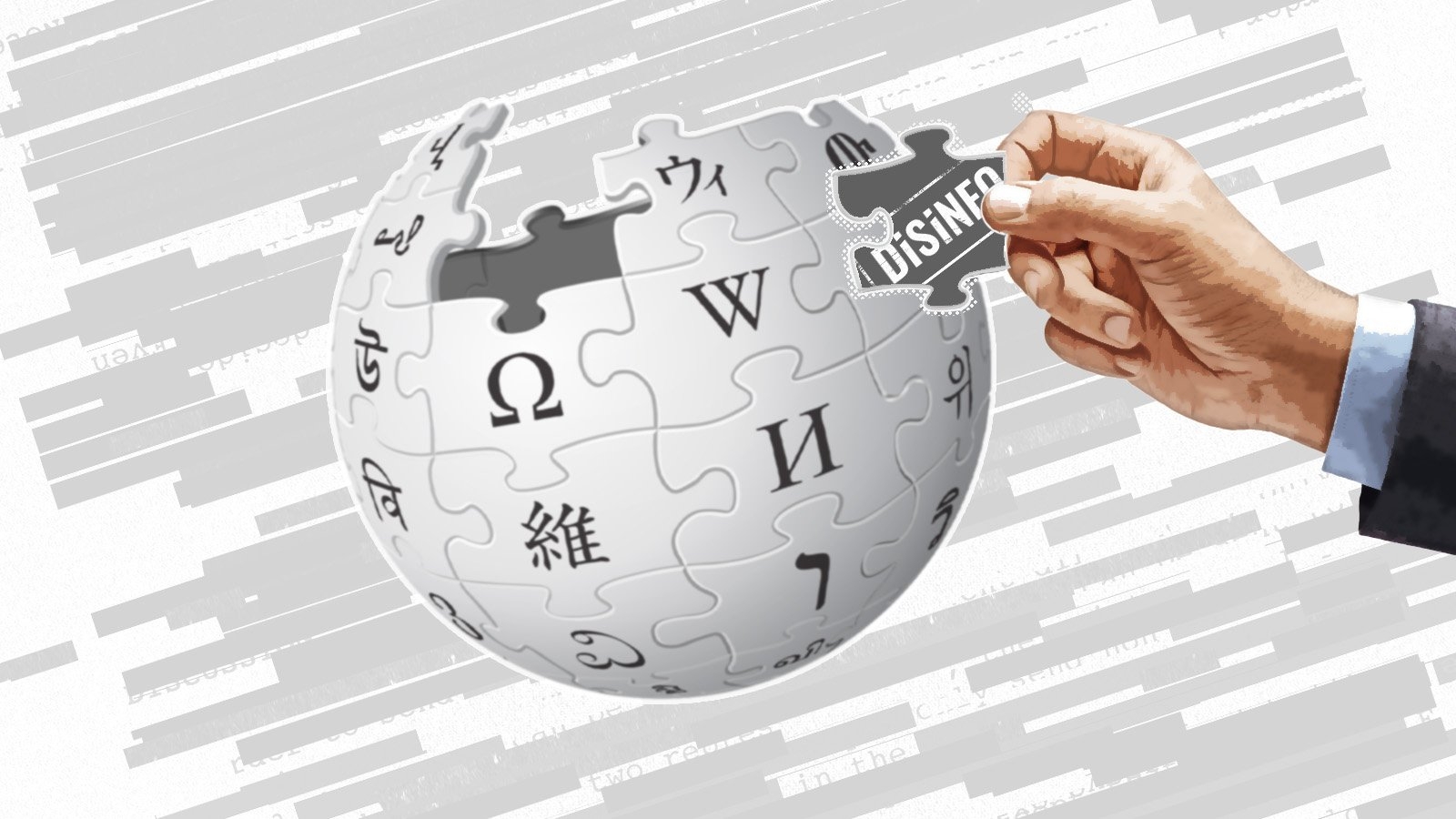
This article has multiple issues. Please help improve it or discuss these issues on the talk page. (Learn how and when to remove these messages)
|
In statistics, probability theory, and information theory, a statistical distance quantifies the distance between two statistical objects, which can be two random variables, or two probability distributions or samples, or the distance can be between an individual sample point and a population or a wider sample of points.
A distance between populations can be interpreted as measuring the distance between two probability distributions and hence they are essentially measures of distances between probability measures. Where statistical distance measures relate to the differences between random variables, these may have statistical dependence, and hence these distances are not directly related to measures of distances between probability measures. Again, a measure of distance between random variables may relate to the extent of dependence between them, rather than to their individual values.
Many statistical distance measures are not metrics, and some are not symmetric. Some types of distance measures, which generalize squared distance, are referred to as (statistical) divergences.
Terminology
Many terms are used to refer to various notions of distance; these are often confusingly similar, and may be used inconsistently between authors and over time, either loosely or with precise technical meaning. In addition to "distance", similar terms include deviance, deviation, discrepancy, discrimination, and divergence, as well as others such as contrast function and metric. Terms from information theory include cross entropy, relative entropy, discrimination information, and information gain.
Distances as metrics
Metrics
A metric on a set X is a function (called the distance function or simply distance) d : X × X → R+ (where R+ is the set of non-negative real numbers). For all x, y, z in X, this function is required to satisfy the following conditions:
- d(x, y) ≥ 0 (non-negativity)
- d(x, y) = 0 if and only if x = y (identity of indiscernibles. Note that condition 1 and 2 together produce positive definiteness)
- d(x, y) = d(y, x) (symmetry)
- d(x, z) ≤ d(x, y) + d(y, z) (subadditivity / triangle inequality).
Generalized metrics
Many statistical distances are not metrics, because they lack one or more properties of proper metrics. For example, pseudometrics violate property (2), identity of indiscernibles; quasimetrics violate property (3), symmetry; and semimetrics violate property (4), the triangle inequality. Statistical distances that satisfy (1) and (2) are referred to as divergences.
Statistically close
The total variation distance of two distributions and
over a finite domain
, (often referred to as statistical difference or statistical distance in cryptography) is defined as
.
We say that two probability ensembles and
are statistically close if
is a negligible function in
.
Examples
Metrics
- Total variation distance (sometimes just called "the" statistical distance)
- Hellinger distance
- Lévy–Prokhorov metric
- Wasserstein metric: also known as the Kantorovich metric, or earth mover's distance
- Mahalanobis distance
- Amari distance
- Integral probability metrics generalize several metrics or pseudometrics on distributions
Divergences
- Kullback–Leibler divergence
- Rényi divergence
- Jensen–Shannon divergence
- Bhattacharyya distance (despite its name it is not a distance, as it violates the triangle inequality)
- f-divergence: generalizes several distances and divergences
- Discriminability index, specifically the Bayes discriminability index, is a positive-definite symmetric measure of the overlap of two distributions.
See also
- Probabilistic metric space
- Randomness extractor
- Similarity measure
- Zero-knowledge proof
Notes
- Dodge, Y. (2003)—entry for distance
- Goldreich, Oded (2001). Foundations of Cryptography: Basic Tools (1st ed.). Berlin: Cambridge University Press. p. 106. ISBN 0-521-79172-3.
- Reyzin, Leo. (Lecture Notes) Extractors and the Leftover Hash Lemma
External links
- Distance and Similarity Measures (Wolfram Alpha)
References
- Dodge, Y. (2003) Oxford Dictionary of Statistical Terms, OUP. ISBN 0-19-920613-9
This article has multiple issues Please help improve it or discuss these issues on the talk page Learn how and when to remove these messages This article includes a list of general references but it lacks sufficient corresponding inline citations Please help to improve this article by introducing more precise citations February 2012 Learn how and when to remove this message This article needs additional citations for verification Please help improve this article by adding citations to reliable sources Unsourced material may be challenged and removed Find sources Statistical distance news newspapers books scholar JSTOR December 2020 Learn how and when to remove this message Learn how and when to remove this message In statistics probability theory and information theory a statistical distance quantifies the distance between two statistical objects which can be two random variables or two probability distributions or samples or the distance can be between an individual sample point and a population or a wider sample of points A distance between populations can be interpreted as measuring the distance between two probability distributions and hence they are essentially measures of distances between probability measures Where statistical distance measures relate to the differences between random variables these may have statistical dependence and hence these distances are not directly related to measures of distances between probability measures Again a measure of distance between random variables may relate to the extent of dependence between them rather than to their individual values Many statistical distance measures are not metrics and some are not symmetric Some types of distance measures which generalize squared distance are referred to as statistical divergences TerminologyMany terms are used to refer to various notions of distance these are often confusingly similar and may be used inconsistently between authors and over time either loosely or with precise technical meaning In addition to distance similar terms include deviance deviation discrepancy discrimination and divergence as well as others such as contrast function and metric Terms from information theory include cross entropy relative entropy discrimination information and information gain Distances as metricsMetrics A metric on a set X is a function called the distance function or simply distance d X X R where R is the set of non negative real numbers For all x y z in X this function is required to satisfy the following conditions d x y 0 non negativity d x y 0 if and only if x y identity of indiscernibles Note that condition 1 and 2 together produce positive definiteness d x y d y x symmetry d x z d x y d y z subadditivity triangle inequality Generalized metrics Many statistical distances are not metrics because they lack one or more properties of proper metrics For example pseudometrics violate property 2 identity of indiscernibles quasimetrics violate property 3 symmetry and semimetrics violate property 4 the triangle inequality Statistical distances that satisfy 1 and 2 are referred to as divergences Statistically closeThe total variation distance of two distributions X displaystyle X and Y displaystyle Y over a finite domain D displaystyle D often referred to as statistical difference or statistical distance in cryptography is defined as D X Y 12 a D Pr X a Pr Y a displaystyle Delta X Y frac 1 2 sum alpha in D Pr X alpha Pr Y alpha We say that two probability ensembles Xk k N displaystyle X k k in mathbb N and Yk k N displaystyle Y k k in mathbb N are statistically close if D Xk Yk displaystyle Delta X k Y k is a negligible function in k displaystyle k ExamplesMetrics Total variation distance sometimes just called the statistical distance Hellinger distance Levy Prokhorov metric Wasserstein metric also known as the Kantorovich metric or earth mover s distance Mahalanobis distance Amari distance Integral probability metrics generalize several metrics or pseudometrics on distributionsDivergences Kullback Leibler divergence Renyi divergence Jensen Shannon divergence Bhattacharyya distance despite its name it is not a distance as it violates the triangle inequality f divergence generalizes several distances and divergences Discriminability index specifically the Bayes discriminability index is a positive definite symmetric measure of the overlap of two distributions See alsoProbabilistic metric space Randomness extractor Similarity measure Zero knowledge proofNotesDodge Y 2003 entry for distance Goldreich Oded 2001 Foundations of Cryptography Basic Tools 1st ed Berlin Cambridge University Press p 106 ISBN 0 521 79172 3 Reyzin Leo Lecture Notes Extractors and the Leftover Hash LemmaExternal linksDistance and Similarity Measures Wolfram Alpha ReferencesDodge Y 2003 Oxford Dictionary of Statistical Terms OUP ISBN 0 19 920613 9